The 39th Annual AAAI Conference on Artificial Intelligence
February 25 – March 4, 2025 | Philadelphia, Pennsylvania, USA
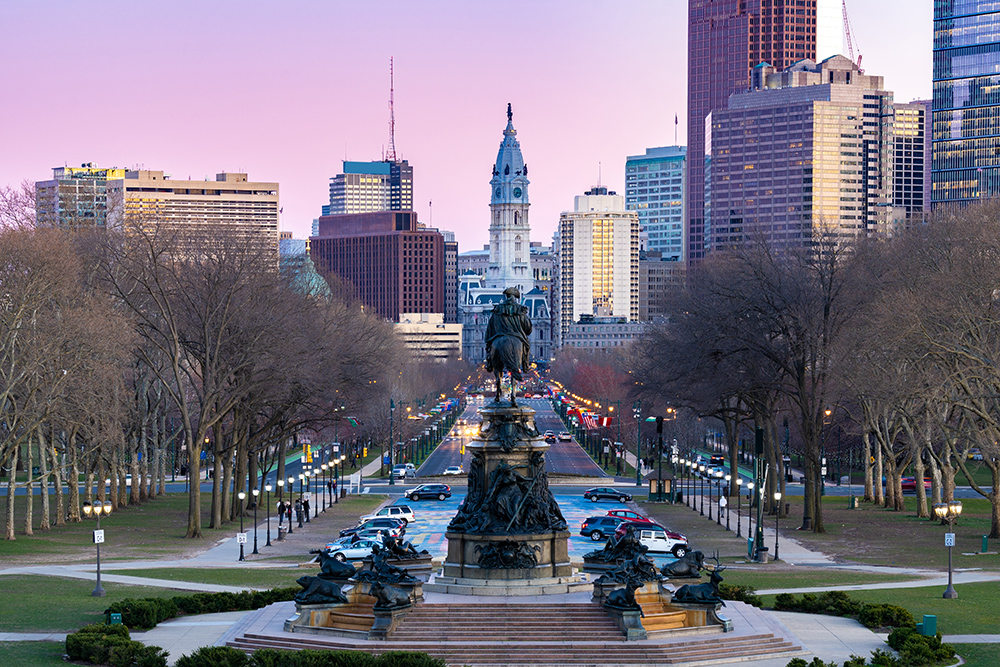
AAAI-25/IAAI-25/EAAI-25 Invited Speakers
Sponsored by the Association for the Advancement of Artificial Intelligence
February 27 – March 2, 2025 | Pennsylvania Convention Center | Philadelphia, PA, USA
Thursday, February 27
8:30AM – 9:25AM | Terrace Ballroom II-IV, 400 Level
Welcome, Opening Remarks, AAAI Awards, and Conference Awards
5:35PM – 6:30PM | Terrace Ballroom II-IV, 400 Level
AAAI Invited Talk
Predicting Career Transitions and Estimating Wage Disparities Using Foundation Models, Susan Athey
Friday, February 28
8:30AM – 9:25AM | Terrace Ballroom II-IV, 400 Level
AAAI/IAAI Invited Talk
AI, Agents and Applications, Andrew Ng
5:35PM – 7:30PM | Terrace Ballroom II-IV, 400 Level
AAAI Invited Talk
Can Large Language Models Reason about Spatial Information?, Tony Cohn
2025 Robert S. Engelmore Memorial Lecture Award Talk
Democratizing AI through Community Organizing, Christoph Schuhmann
Saturday, March 1
8:30AM – 9:25AM | Terrace Ballroom II-IV, 400 Level
AAAI Invited Talk
Algorithmic Agnotology, Alondra Nelson
2:00PM – 3:15PM | Room 113A, 100 Level, East Building
AAAI/EAAI Patrick Henry Winston Outstanding Educator Award
T(w)eaching AI in the Age of LLMs, Subbarao Kambhampati
4:15PM – 5:15PM | Terrace Ballroom II-IV, 400 Level
Presidential Address
AI Reasoning and System 2 Thinking, Francesca Rossi
5:15PM – 5:45PM | Terrace Ballroom II-IV, 400 Level
Presidential Panel on the Future of AI Research, Francesca Rossi
5:45PM – 6:30PM | Terrace Ballroom II-IV, 400 Level
Annual Meeting of AAAI Members
Sunday, March 2
8:30AM – 9:25AM | Terrace Ballroom II-IV, 400 Level
AI for Humanity Award
Can AI Benefit Humanity?, Stuart J. Russell
5:05PM – 6:00PM | Terrace Ballroom II-IV, 400 Level
AAAI Invited Talk
Propositional Interpretability in Humans and AI Systems, David Chalmers
AAAI Invited Talk
Predicting Career Transitions and Estimating Wage Disparities Using Foundation Models
Susan Athey
This talk focuses on the use of foundation models to study problems in labor economics, in particular problems relating to the progression of worker careers and wages. In Vafa et al. (2024a), we introduced a transformer-based predictive model, CAREER, that predicts a worker’s next job as a function of career history (an “occupation model”). CAREER was initially estimated (“pre-trained”) using a large, unrepresentative resume dataset, which served as a “foundation model,” and parameter estimation was continued (“fine-tuned”) using data from a representative survey. CAREER had better predictive performance than benchmarks. Athey et al (2024) considers an alternative where the resume-based foundation model is replaced by a large language model (LLM). We convert tabular data from the survey into text files that resemble resumes and fine-tune the LLMs using these text files with the objective to predict the next token (word). The resulting fine-tuned LLM is used as an input to an occupation model. Its predictive performance surpasses all prior models. We demonstrate the value of fine-tuning and further show that by adding more career data from a different population, fine-tuning smaller LLMs surpasses the performance of fine-tuning larger models. In Vafa et al. (2024b), we apply and adapt this framework to the problem of gender wage decompositions, which require estimating the portion of the gender wage gap explained by career histories of workers. Classical methods for decomposing the wage gap employ simple linear models, and the resulting decompositions thus suffer from omitted variable bias (OVB), where covariates that are correlated with both gender and wages are not included in the model. We explore an alternative methodology for wage gap decomposition that employs CAREER as a foundation model. We prove that the way foundation models are usually trained might still lead to OVB, but develop fine-tuning algorithms that empirically mitigate this issue. We first provide a novel set of conditions under which an estimator of the wage gap based on a fine-tuned foundation model is root-n-consistent. Building on the theory, we then propose methods for fine-tuning foundation models that minimize OVB. Using data from the Panel Study of Income Dynamics, we find that history explains more of the gender wage gap than standard econometric models can measure, and we identify elements of history that are important for reducing OVB.
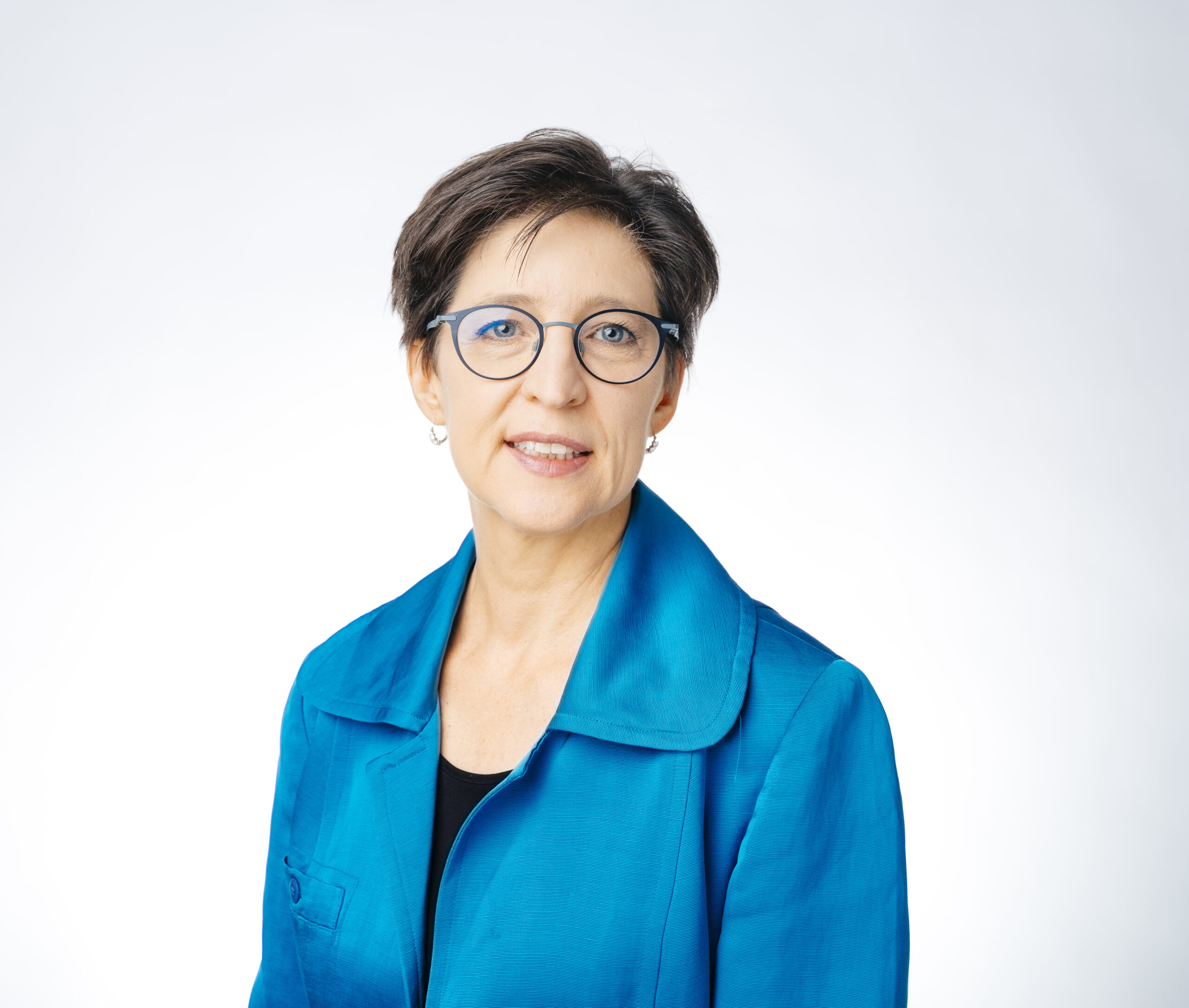
Professor Athey is the Economics of Technology Professor at Stanford Graduate School of Business. She received her bachelor’s degree from Duke University and her PhD from Stanford, and she holds an honorary doctorate from Duke University. She previously taught at the economics departments at MIT, Stanford, and Harvard. She is an elected member of the National Academy of Science and is the recipient of the John Bates Clark Medal, awarded by the American Economics Association to the economist under 40 who has made the greatest contributions to thought and knowledge. Her current research focuses on the economics of digitization, marketplace design, and the intersection of econometrics and machine learning. She has worked on several application areas, including timber auctions, internet searches, online advertising, the news media, and social impact applications of digital technology. As one of the first “tech economists,” she served as consulting chief economist for Microsoft Corporation for six years and has served on the boards of multiple private and public technology firms. She also served as a long-term advisor to the British Columbia Ministry of Forests, helping architect and implement their auction-based pricing system. She was a founding associate director of the Stanford Institute for Human-Centered Artificial Intelligence, and she is the founding director of the Golub Capital Social Impact Lab at Stanford GSB. She served two years as Chief Economist at the U.S. Department of Justice Antitrust Division. Professor Athey was the 2023 President of the American Economics Association, where she previously served as vice president and elected member of the Executive Committee.
AAAI/IAAI Invited Talk
AI, Agents and Applications
Andrew Ng
In this keynote, Andrew will explore how current technologies, particularly agentic workflow, are revolutionizing the development of AI products. He will also delve into the role of AI in prototyping, showcasing how AI lowers the cost of software development and accelerates the prototyping process.
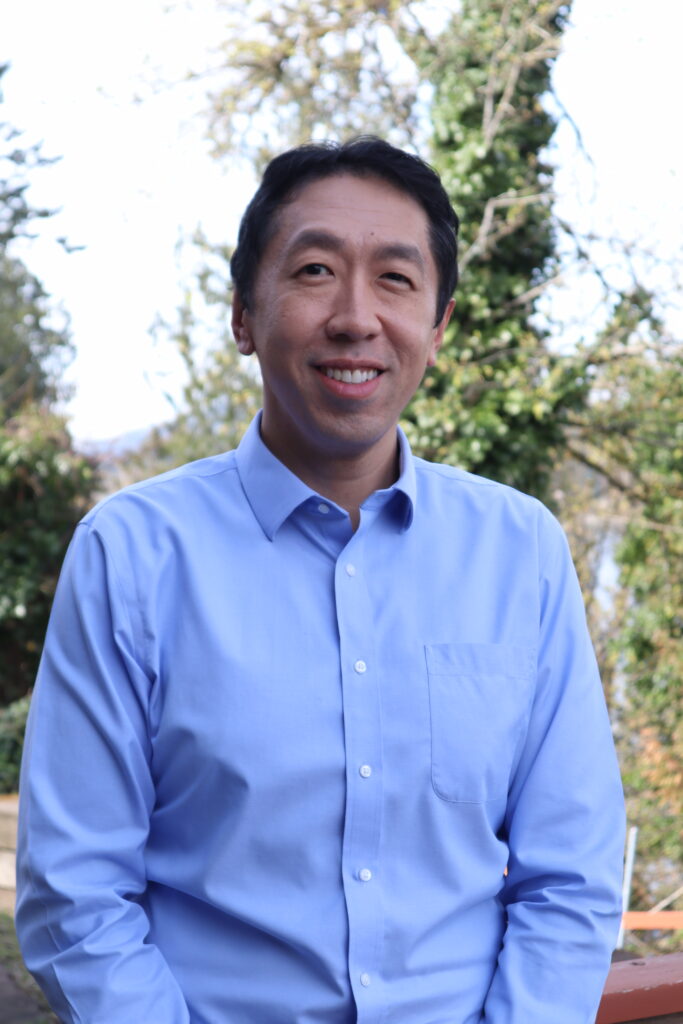
Andrew Ng is the Founder of DeepLearning.AI, Managing General Partner at AI Fund, Exec Chairman of LandingAI, Chairman and Co-Founder of Coursera, and an Adjunct Professor at Stanford University. As a pioneer in machine learning and online education, Dr. Ng has changed countless lives through his work in AI. Over 8 million people have taken an AI class from him. He was the founding lead of the Google Brain team, which helped Google transform into a modern AI company. He served as VP & Chief Scientist at Baidu, where he led a 1,300-person AI team responsible for the company’s AI technology and strategy. He was formerly Director of the Stanford AI Lab, home to 20+ faculty members and research groups. In 2023, he was named to the Time100 AI list of the most influential AI persons in the world. Dr. Ng now focuses his time primarily on providing AI training and on his entrepreneurial ventures, looking for the best ways to accelerate responsible AI adoption globally.
Dr. Ng has authored over 200 papers in AI and related fields, and holds a B.Sc. from CMU, M.Sc. from MIT and Ph.D. from UC Berkeley.
He also serves on Amazon’s board of directors.
AAAI Invited Talk
Can Large Language Models Reason about Spatial Information?
Tony Cohn
Spatial reasoning is a core component of an agent’s ability to operate in or reason about the physical world. LLMs are widely promoted as having abilities to reason about a wide variety of domains, including commonsense. In this talk I will discuss the ability of state-of-the-art LLMs to perform commonsense reasoning, particularly with regard to spatial information. Across a wide range of LLMs, although they show abilities rather better than chance, they still struggle with many questions and tasks, for example when reasoning about directions, or topological relations.
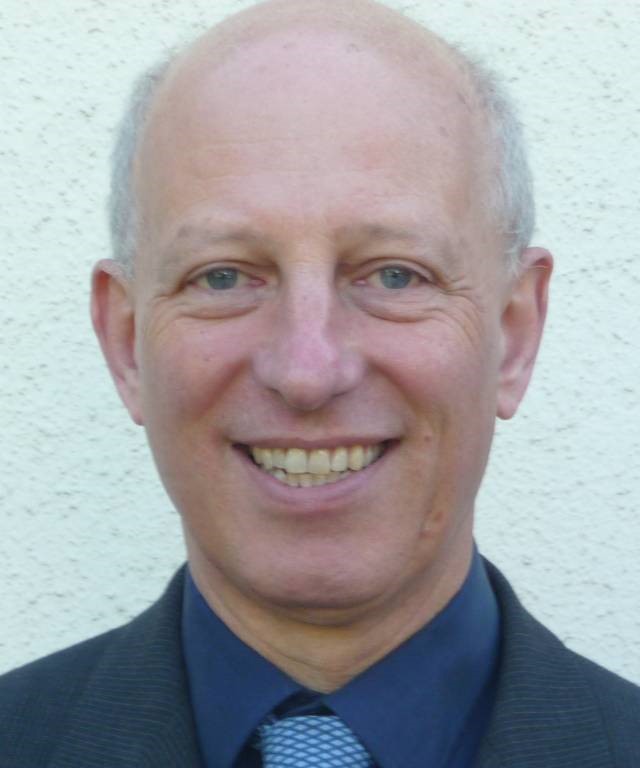
Anthony (Tony) Cohn is Professor of Automated Reasoning in the School of Computing, University of Leeds. His current research interests range from theoretical work on spatial calculi (receiving a KR test-of-time classic paper award in 2020) and spatial ontologies, to cognitive vision, modelling spatial information in the hippocampus, and Decision Support Systems, particularly for the built environment, as well as robotics. He is Foundation Models lead at the Alan Turing Institute where he is conducting research on evaluating the capabilities of large language models, in particular with respect to commonsense reasoning. He is Editor-in-Chief of Spatial Cognition and Computation and was previously Editor-in-chief of the AI journal. He is the recipient of the 2021 Herbert A Simon Cognitive Systems Prize, as well as Distinguished Service Awards from AAAI, IJCAI, KR and EurAI. He is a Fellow of the Royal Academy of Engineering, and of the AI societies AAAI, AISB, EurAI and AAIA.
2025 Robert S. Engelmore Memorial Lecture Award Talk
Democratizing AI through Community Organizing
Christoph Schuhmann
This talk explores LAION’s journey—a non-profit born from a grassroots online community of students, programmers, researchers, and enthusiasts—united by the vision of democratizing AI. Through collaborative efforts, LAION provides free access to powerful tools, empowering individuals worldwide to contribute to and benefit from groundbreaking AI advancements.
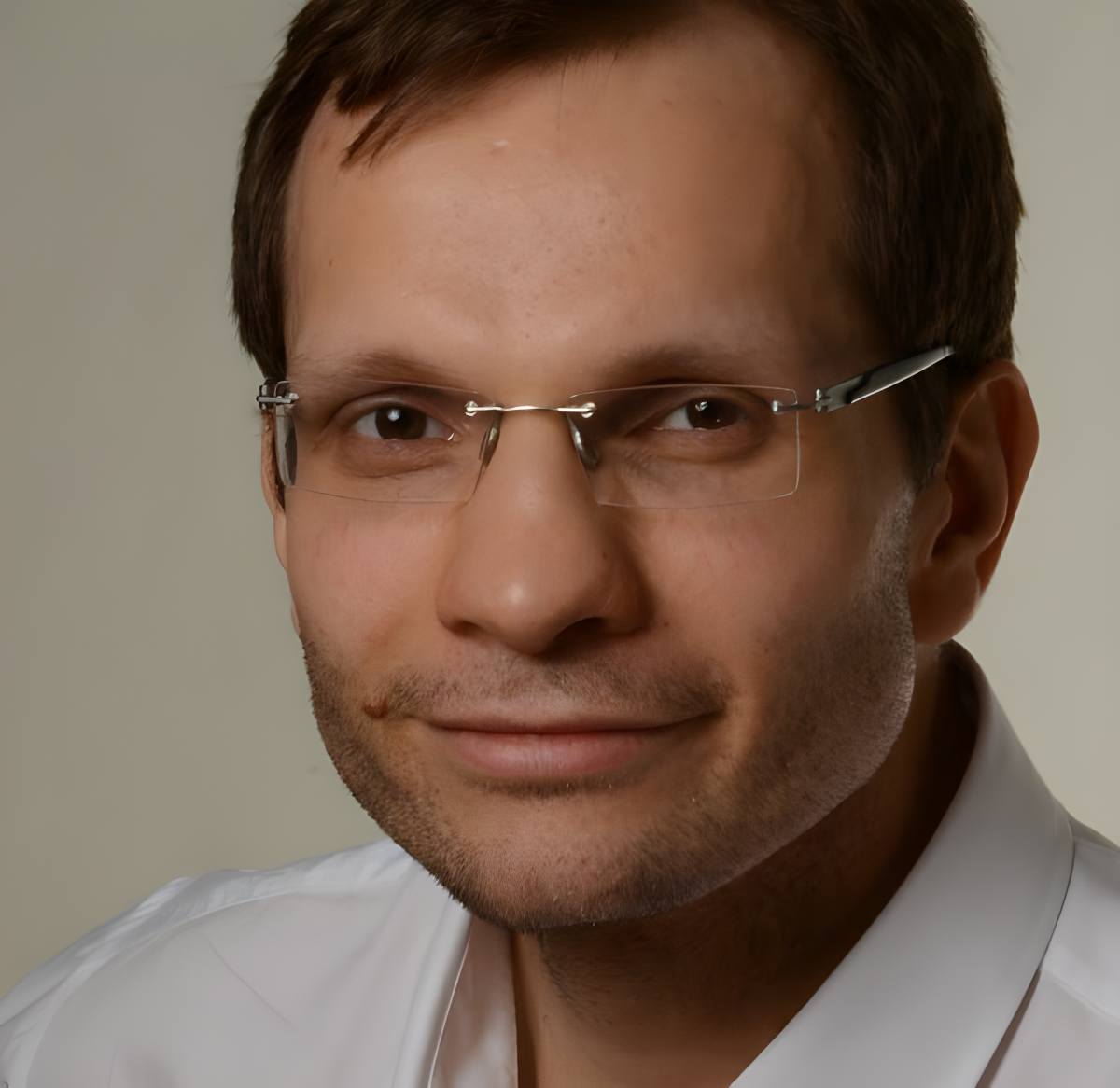
Christoph Schuhmann, an educator and hobby scientist from Germany, is the co-founder of LAION, a non-profit dedicated to democratizing AI through open-source datasets and tools. Under his leadership, LAION developed pivotal datasets like LAION-5B and LAION-400M, which have powered renowned AI models including OpenCLIP, Stable Diffusion, and Google’s Imagen. Schuhmann also initiated the OpenAssistant project with Yannic Kilcher and Andreas Köpf, which pioneered the creation of open-source instruction tuning datasets for the first ChatGPT-like source models.
AAAI Invited Talk
Algorithmic Agnotology
Alondra Nelson
Agnotology comes from the study of ignorance and refers to active denial through, frequently, disinformation. Though the term has been applied to the tobacco industry and climate change, Dr. Nelson uses the term to reference the obscuration of AI systems and argues that there is a similar denial of the harms and risks of today’s AI systems.
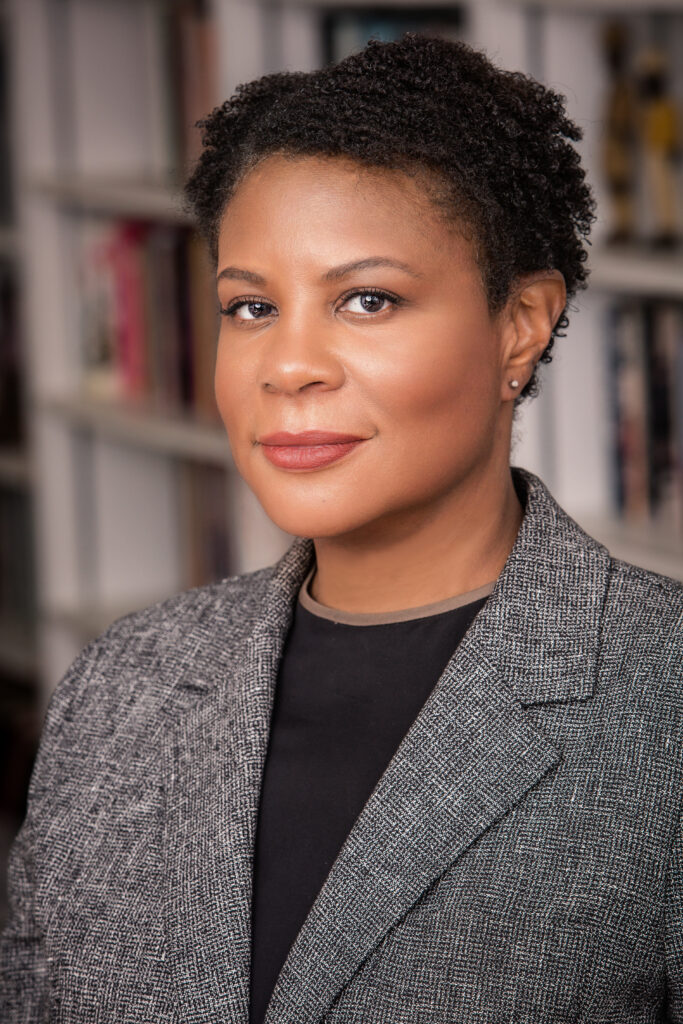
Dr. Alondra Nelson holds the Harold F. Linder Chair in the School of Social Science at the Institute of Advanced Study in Princeton, New Jersey, where she also founded and leads the Science, Technology, and Social Values Lab. From 2021 to 2023, she was deputy assistant to President Joe Biden and acting director and the first person to serve as principal deputy director for science and society of the White House Office of Science and Technology Policy (OSTP). She led the development of the White House “Blueprint for an AI Bill of Rights,” a cornerstone of President Biden’s Executive Order on the Safe, Secure, and Trustworthy Development and Use of Artificial Intelligence. In recognition of Nelson’s impactful OSTP tenure, Nature named her to its global list of the 10 People Who Shaped Science. In 2023, she was included in the inaugural TIME100 list of the most influential people in AI, and was nominated by the White House and then appointed by United Nations Secretary-General António Guterres to serve on the UN High-level Advisory Body on Artificial Intelligence. In 2024, Nelson was appointed by President Biden to the National Science Board, the body that establishes the policies of the National Science Foundation and advises Congress and the President. Nelson previously was Columbia University’s first Dean of Social Science and served as the 14th president and CEO of the Social Science Research Council, an independent, international nonprofit organization.
AAAI/EAAI Patrick Henry Winston Outstanding Educator Award
T(w)eaching AI in the Age of LLMs
Subbarao Kambhampati
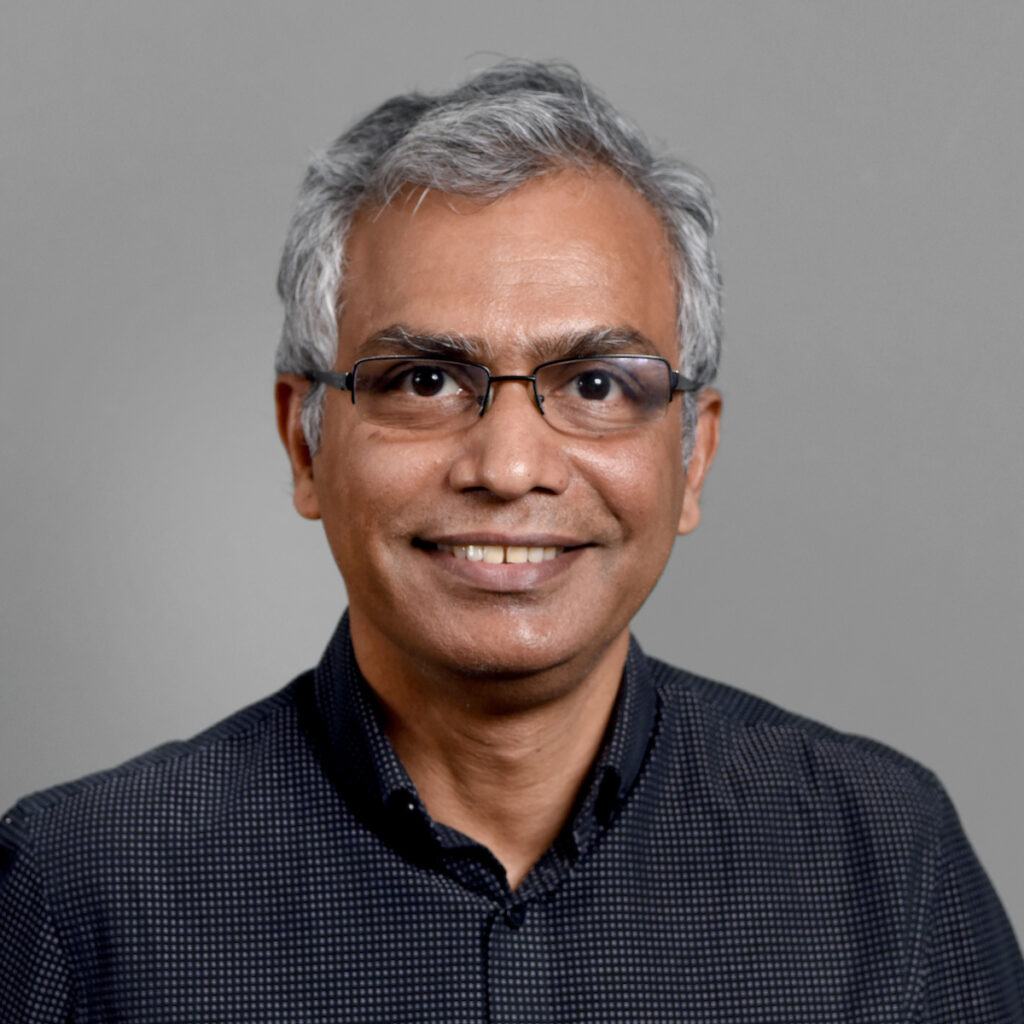
Subbarao Kambhampati is a professor of computer science at Arizona State University. Kambhampati studies fundamental problems in planning and decision making, motivated in particular by the challenges of human-aware AI systems. He is a fellow of AAAI, AAAS and ACM. He served as the president of the Association for the Advancement of Artificial Intelligence, a trustee of the International Joint Conference on Artificial Intelligence, the chair of AAAS Section T (Information, Communication and Computation), and was a founding board member of Partnership on AI. Kambhampati’s research as well as his views on the progress and societal impacts of AI have been featured in multiple national and international media outlets. He can be followed on Twitter @rao2z.
AAAI Presidential Address
Francesca Rossi
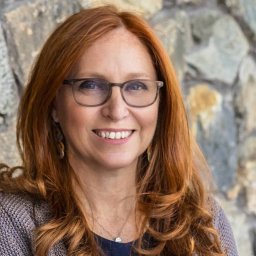
Francesca Rossi is an IBM fellow and the IBM AI Ethics Global Leader. She works at the T.J. Watson IBM Research Lab, New York. Her research interests focus on artificial intelligence, specifically they include constraint reasoning, preferences, multi-agent systems, computational social choice, and collective decision making. She is also interested in ethical issues in the development and behaviour of AI systems, in particular for decision support systems for group decision making. She has published over 200 scientific articles in journals and conference proceedings, and as book chapters. She has co-authored a book and she has edited 17 volumes, between conference proceedings, collections of contributions, special issues of journals, and a handbook.
AI for Humanity Award
Can AI Benefit Humanity?
Stuart J. Russell
The media are agog with claims that recent advances in AI put artificial general intelligence (AGI) within reach. Is this true? If so, is that a good thing? Alan Turing predicted that AGI would result in the machines taking control. Turing was right to express concern but wrong to think that doom is inevitable. Yet the question of whether superior AI systems can really benefit humanity remains unanswered. It may be that they can do so only by their absence.
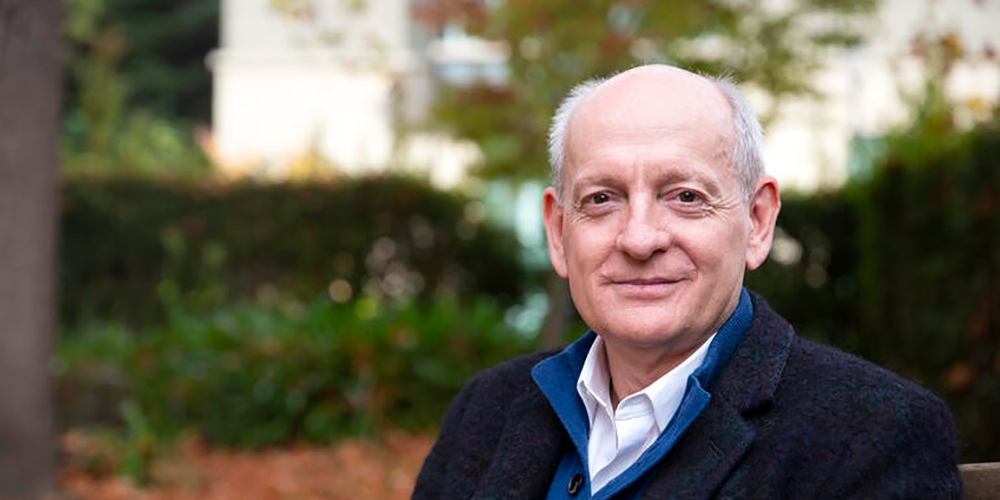
Stuart Russell is the Michael H. Smith and Lotfi A. Zadeh Chair in Engineering and a professor in the Division of Computer Science, EECS. His research covers a wide range of topics in artificial intelligence including machine learning, probabilistic reasoning, knowledge representation, planning, real-time decision making, multitarget tracking, computer vision, computational physiology, and philosophical foundations. He has also worked with the United Nations to create a new global seismic monitoring system for the Comprehensive Nuclear-Test-Ban Treaty. His current concerns include the threat of autonomous weapons and the long-term future of artificial intelligence and its relation to humanity. The latter topic is the subject of his new book, “Human Compatible: AI and the Problem of Control” (Viking/Penguin, 2019), which was excerpted in the New York Times and listed among Best Books of 2019 by the Guardian, Forbes, the Daily Telegraph, and the Financial Times.
AAAI Invited Talk
Propositional Interpretability in Humans and AI Systems
David Chalmers
Mechanistic interpretability is one of the most exciting and important research programs in current AI. My aim is to build some philosophical foundations for the program, along with setting out some concrete challenges and assessing progress to date. I will argue for the importance of propositional interpretability, which involves interpreting a system’s mechanisms and behavior in terms of propositional attitudes: attitudes (such as belief, desire, or subjective probability) to propositions (e.g. the proposition that it is hot outside). Propositional attitudes are the central way that we interpret and explain human beings and they are likely to be central in AI too. A central challenge is what I call *thought logging*: creating systems log all of the relevant propositional attitudes in an AI system over time. I will examine currently popular methods of interpretability (such as probing, sparse auto-encoders, and chain of thought methods) as well as philosophical methods of interpretation (including psychosemantics and representation theorems) to assess their strengths and weaknesses as methods of propositional interpretability.
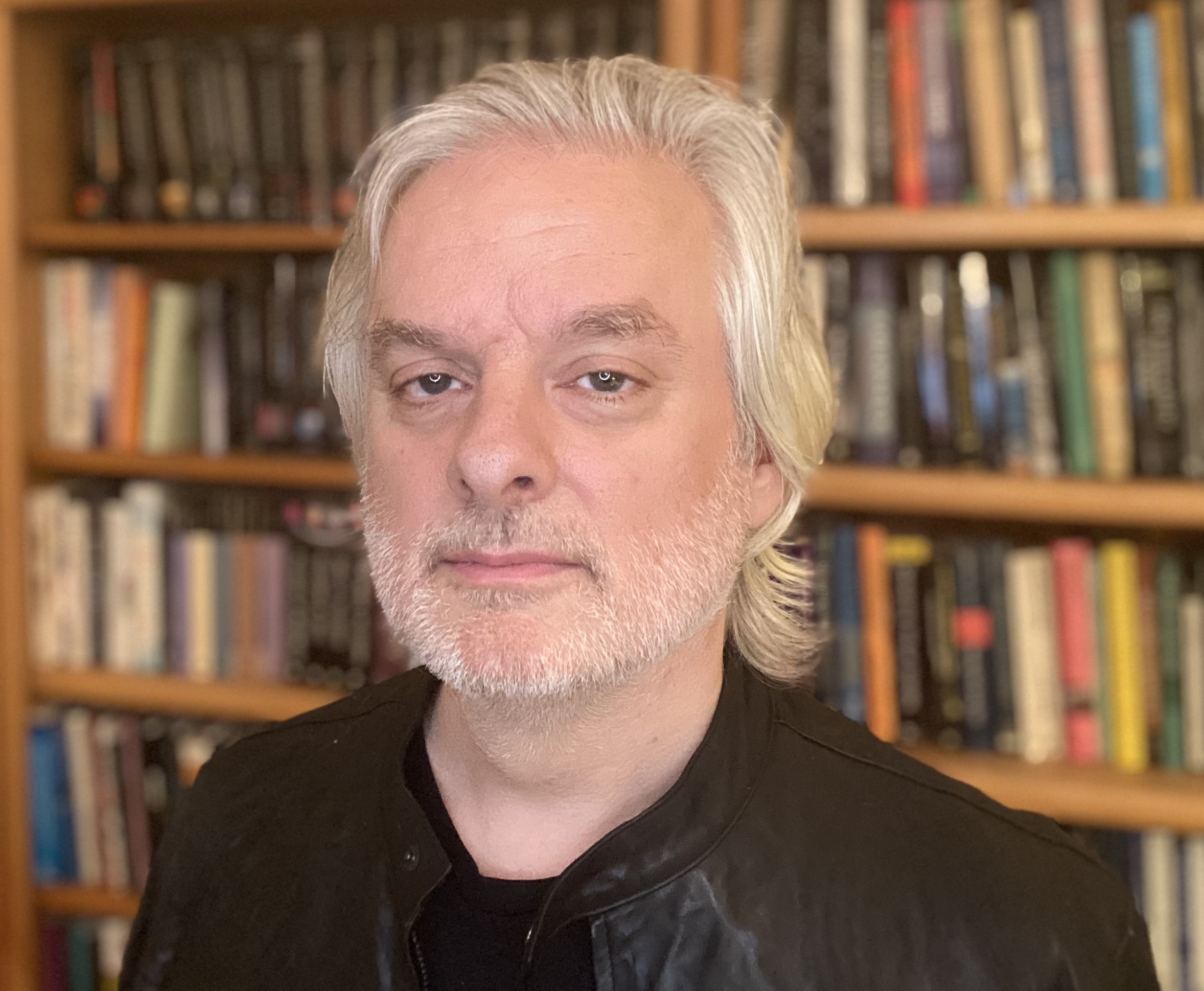
David Chalmers is University Professor of Philosophy and Neural Science and co-director of the Center for Mind, Brain, and Consciousness at New York University. He is the author of The Conscious Mind (1996), Constructing the World (2012), and Reality+ (2022). He is a past president of both the American and Australian Philosophical Associations as well as the Association for the Scientific Study of Consciousness. He received his Ph.D. in Philosophy and Cognitive Science from Indiana in 1993, advised by Douglas Hofstadter. He is known for formulating the “hard problem” of consciousness, which inspired Tom Stoppard’s play The Hard Problem; for the idea of the “extended mind,” which says that the tools we use can become parts of our minds; and for work on foundational issues in AI, including language and learning in artificial neural networks.